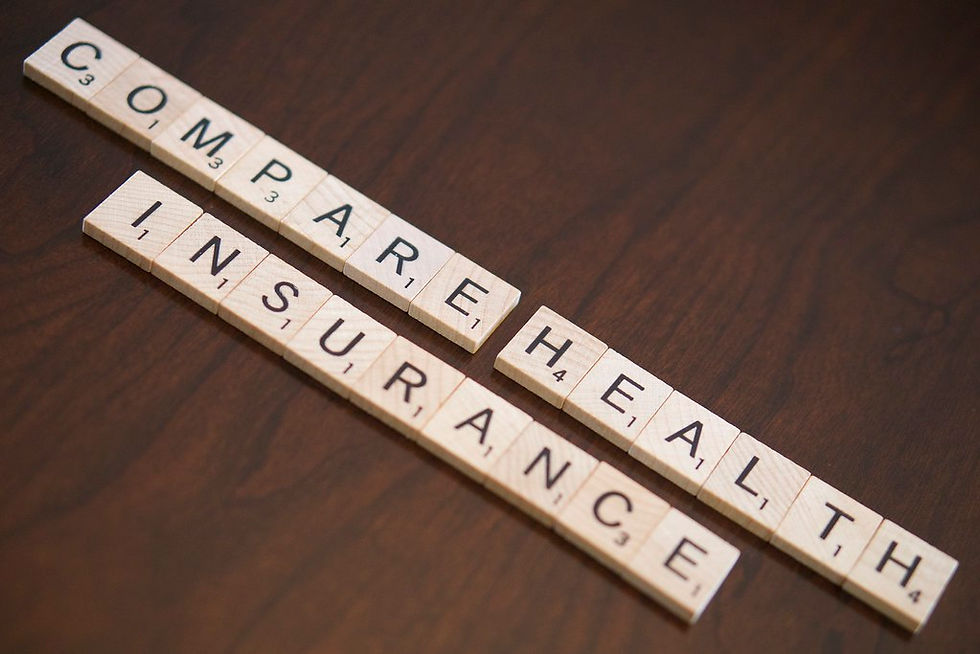
An Analysis of Uninsurance Rates Among
Healthcare Personnel and Associated Losses
From COVID-19
Analyzing the rates of HCP uninsurance in the context of the pandemic and forecasting associated losses in upcoming years.
In the United States, healthcare insurance is considered to be the main method of affording health services, for both unexpected and preventative care [1]. Numerous studies have detailed the importance of healthcare insurance, as uninsured individuals are known to have less access to care, receive poorer quality of care, and even experience worse health outcomes than that of their insured counterparts [2]. Though national uninsurance trends are fairly well studied, rates of uninsurance within healthcare personnel (HCP) receive much less attention. According to data from the American Community Survey in 2018, approximately 600,000 healthcare workers were uninsured [3]. In the context of the COVID-19, HCP are known to be at a much higher risk of exposure and infection in comparison to the general population. Evidently, investigating the loss of life and health insurance costs from COVID-19 among uninsured
populations of HCP is important to understanding the impact of the pandemic on this population.
The main objective of our study is to evaluate trends in uninsurance among HCP and investigate the resulting healthcare costs associated with this population as a result of COVID-19. This mainly consists of predictive models for treatments, various calculations for losses, and trend models to predict rates of uninsurance in current and future years. We utilized data from two main sources in our modeling: The Census Bureau’s American Community Survey’s PUMS Database, and the COVID-19 Restricted Access Surveillance Data provided by The Center for Disease Control. We also utilized the cost of treatment information from Peterson-KFF Briefs to perform loss calculations, as well as from other credible online sources. We produced a total of six binary classification models, three of which were specific to Healthcare Personnel and three of which were for the general population (all cases). We were able to predict HCP mechanical ventilator use with an accuracy of approximately 92% and HCP ICU Admission with an accuracy of approximately 92%. The hospital admission was predicted with an accuracy of approximately 67%. In the trend analysis, we performed a polynomial regression on two sets of points: the uninsured population of HCP, and the total population of HCP. Our forecasting predicted 1,519,533 uninsured HCP in the year 2020, and 21,536,576 total HCP in the year 2020. This resulted in an uninsurance rate of approximately 7.1% among all HCP in 2020. Using our predictive models, we found that approximately 74 million dollars in losses were incurred from treatment of HCP.
Overall, increasing the insured population of HCP not only benefits them, but also those who they provide care to. Creating and implementing these policies is important, especially under circumstances of infectious disease. Although we may have possibly incurred millions of dollars’ worth of healthcare losses from uninsured HCP in COVID-19, this scenario does not have to repeat itself. In the future, implementing the policies and making decisions as detailed above lessen the extent of a major crisis while allowing HCP and those they work with to be safe in their environments.